What is Hindsight Bias?
Mike Carroll, VP, Corporate Transformation, Strategy Development and Innovation, Georgia Pacific on Hindsight Bias in Workflow Automation
Add bookmark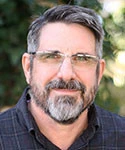
Before you watch Mike Carroll, VP, Corporate Transformation, Strategy Development and Innovation, Georgia Pacific present on Hindsight Bias in Workflow Automation at the Workflow Management LIVE virtual event, read this introduction to hindsight bias and other key areas he will be discussing.
What is Hindsight Bias
They say that hindsight is 20/20, but is it? Humans are hard-wired to try to make sense out of a senseless and chaotic world. Though this tendency has its evolutionary purpose, it does lead to a psychological phenomenon known as hindsight bias: the tendency we all have to convince ourselves after an event that we accurately predicted it before it happened.
As it causes people to become overconfident in their ability to predict outcomes, it can negatively impact decision making as well as lead people to become needlessly frustrated when the unexpected happens (i.e. “I should have seen it coming”). In organizational settings especially, it makes it difficult to differentiate between causation and correlation.
The key to minimizing hindsight bias, according to Mike Carroll, the VP, Corporate Transformation, Strategy Development and Innovation at Georgia Pacific, are structural causal models combined with enterprise domain knowledge.
But wait. What is Enterprise Domain Knowledge?
Successful digital transformations and workflow automation projects don’t happen in a vacuum. They require extensive domain knowledge into your customer, your industry and the internal working of your organization.
In the simplest terms, domain knowledge is specialized knowledge or expertise in one particular area. Though it could be applied in other contexts, “domain knowledge” is primarily used to describe subject matter expertise within business and IT settings. For example an HR analyst could be described as a data scientist with deep HR domain knowledge.
Whether you’re looking to automate a single workflow or creating a long-term digital transformation roadmap, developing a deep domain expertise into whatever area you're trying to transform is critical for success. This usually requires extensive interviews with customers/users and internal stakeholders along with a deep dive into historical data.
And what is a causal model?
According to Stanford’s Encyclopedia of Philosophy, causal models are “mathematical models representing causal relationships within an individual system or population. They facilitate inferences about causal relationships from statistical data. They can teach us a good deal about the epistemology of causation, and about the relationship between causation and probability.”
In other words, while traditional predictive models predict future outcomes based on correlations, causal modeling techniques seek to illuminate cause-and-effect relationships. This knowledge helps businesses better understand how multiple variables within a given domain affect each other and use this insight to enhance decision making.
For example, a regular machine learning model (ML) might predict the likelihood of rain based on the number of clouds in the sky. On the other hand, an ML model with causal reasoning can say whether the clouds actually created the rain and whether an increase of clouds will create more rain.
By revealing how different choices affect probabilities of various outcomes, casual analytics can have tremendous impact on the business. However, to find out more about this, you’ll have to watch Mike Carroll’s Workflow Management session LIVE or ON DEMAND.