What is Scalable RPA & Intelligent Automation?
A quick guide for establishing a vision for scalable RPA & IA
Add bookmark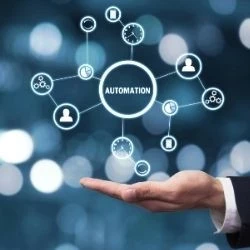
Robotic process automation (RPA) and Intelligent Automation (IA) have proven to be powerful enablers of digital transformation. By automating and digitizing business processes, cognitive technologies not only reduce costs and increase productivity, they also enable organizational agility, facilitate next generation data and analytics capabilities, and boost a company’s overall competitive advantage.
However, despite all of this, 44% of respondents on a recent Bain survey said their automation projects have failed to deliver expected results.
There’s no doubt about it: cognitive technology can be difficult and costly to implement. Even rules-based RPA “bots” can be incredibly expensive to license, test and implement; especially considering some can only be applied to a handful of (or sometimes only one), business processes. Also, given the nature of RPA contracts, companies are often obligated to license a fixed number of bots per year. As a result, companies end up paying for bots they either never or rarely use.
In addition, IA and RPA implementation does not happen in a vacuum. Solutions this sophisticated often require significant upgrades to IT infrastructure as well as the hiring of new, skilled talent and the extensive reskilling of current employees. As a result, the indirect costs of IA implementation alone can easily outweigh the proposed benefits, especially if the solution is only applied to low value processes.
Companies that do achieve optimal results have one thing in common: a clear vision and roadmap for RPA and IA success.
Scaling Robotic process automation (RPA) and Intelligent Automation (IA)
Scalable RPA and IA refers to the strategies, process and technologies involved with:
- deploying RPA and Intelligent Automation across an enterprise
- ensuring RPA and Intelligent Automation solutions are agile enough to accommodate growth and rapid change
- creating and communicating a shared vision of success
Generally speaking, the goal of scalable RPA and IA is not only to ensure that these tools can not only handle spikes in workload and expand scope of usage, but establish one, enterprise-wide approach to implementing, leveraging and capturing value from automation.
Achieving scalability is important for a number of reasons. To start, as any business school freshman knows, increasing economies of scale boosts profitability. The more people who use RPA and IA, the lower per-unit costs will be.
Furthermore, historically speaking, many organizations have embraced a factory-like approach to automation. In other words, they start small, essentially piloting RPA or similar technologies on one process at a time. However, this approach rarely delivers desired results for a number reasons. For starters, if you only automate simple, low value processes, no one is going to be wowed by the results. Secondly, if every function or team is left to their own devices, you’ll end up with hundreds of overlapping but disparate solutions that may perform their given task well enough, but don’t work together, share information or serve any real unified purpose.
Leading edge companies are realizing that in order for IA and RPA to deliver transformational results, these tools must be part of broader, enterprise-wide digital transformation strategy. Instead of experimenting with intelligent solutions the old fashioned way, the idea is to skip the piloting phase all together and go from vision to scale. The first step? Outlining what you want automation to achieve.
Establishing an enterprise-wide strategy for intelligent automation.
Instead of “experimenting” with RPA & IA in an ad hoc fashion, those who have successfully scaled these technologies have first established and communicated a comprehensive, enterprise-wide vision for what they wanted automation to achieve. This shared roadmap to success should clearly communicate what needs to be done (i.e. process to be optimized, legacy systems that need to be updated), how (i.e. what technologies to use, integration strategy, etc.) and why (i.e. how will automation will deliver value and boost competitive advantage). The strategy should also clearly define KPIs, responsibilities and standards.
Author and AI expert Seth Earley encourages organizations to create what he calls ontologies; essentially a holistic digital roadmap of every piece of information that matters to the business, from processes to products to people. As he explains, “Without a consistent, thoughtful approach to developing, applying, and evolving an ontology, AI systems can only develop in a piecemeal, fragmented way — they will lack the underpinning that would allow them to be smart enough to make an impact. The ontology, as a representation of what matters within the company and makes it unique, is what unifies those solutions and makes them more than just a quick fix that will just as quickly become obsolete.”
This strategy should also help automation drive organizational change efforts. Afterall, enterprise-wide digital transformation does not only refer to the technology involved, but mindsets as well. According to Mckinsey, scaling intelligent automation requires 3 fundamental shifts:
- transition from siloed work to interdisciplinary collaboration
- switch from experience-based, leader-driven decision making to data-driven decision making
- move from rigid and risk averse to agile, experimental, and adaptable
IT Readiness
Before RPA and IA can be scaled, you have to ensure your IT infrastructure and data management systems are scalable as well. As AI and RPA solutions require massive amounts of computing power and storage capacity, many companies find that their legacy IT environments simply can’t support intelligent automation. As a result, many organizations are looking towards third party providers and cloud technologies, particularly hybrid cloud solutions, to support AI efforts in the most cost effective and efficient way possible.
Deploying MA and AI also requires a well-thought-out workflow that starts that enables data to be properly collected, cleaned and labeled in order to be “discovered” by the features used for prediction or decision making. Edge analytics enables you to create tiers of data service where you can prioritize data to be passed down the pipeline to feed your AI applications
Automation Center of Excellence (CoE)
Automation Center of Excellence (CoE) is a centralized unit tasked with overseeing the deployment, management, and expansion of intelligent automation across the entire enterprise. As it consolidates power and decision making into one core group, it can also be a key enabler of scalability.
Automation or RPA CoE are typically responsible for:
- Defining the framework for how automation opportunities are identified and prioritized
- Selecting automation technology, developing tools and standards, managing software licensing and vendor relationships, building security standards and monitoring bots in production
- Establishing governance bodies, determining decision-making rights, defining funding mechanisms, creating standards and controls
- Providing technical tool training, formulating the Organizational Change Management (OCM) methodology, tools and templates
- Defining the service catalog, providing deliverables templates, devising development standards and maintaining bot asset inventory
By consolidating decision making into one focused team, organization can more effectively:
- avoid duplication of effort and expense
- focus on time to market (increase speed of delivery_
- drive business agility
- leverage economies of scale where possible
Image sourced from "DESIGNING THE RIGHT OPERATING MODEL FOR YOUR AUTOMATION CENTER OF EXCELLENCE," https://www.infosysconsultinginsights.com/2018/01/18/automation-centers-of-excellence/
What is Hyperautomation?
A term often associated with scalable RPA & AI, Hyperautomation is, according to Gartner, “the application of advanced technologies, including artificial intelligence (AI) and machine learning (ML), to increasingly automate processes and augment humans.” Rather than referring to any single tool, hyperautomation concerns the development of sophisticated, interoperable technological ecosystems capable of enabling the automation of unstructured, complex processes.
The idea behind hyperautomation is that by constantly increasing automation, processes continuously become more efficient while simultaneously generating more data for fact-based decisions on what to automate next. In other words, hyperautomation self-perpetuates the continuous improvement cycle, delivering more and more value every day.
____________________________
We at ADA are thrilled to announce we're developing new, in-depth, data-based report on scalable RPA and IA. This comprehensive, industry report will take a deep dive into:
- Key factors for achieving scalable RPA & IA
- The key challenges associated with scalable IA and RPA as well as how to overcome them
- How companies are leveraging AI & RPA to to deliver maximum value to the business
Have 2 minutes? Let us know how your organization is approaching scalable RPA and IA.
Create your own user feedback survey
Can't view the survey above? Access it here: https://www.surveymonkey.com/r/JWPFNFL
We respect your privacy, by completing this survey and clicking "Submit" you agree to having your details passed onto the sponsor who may promote similar products and services related to your area of interest subject to their privacy policy. You have the right to object. In addition, you will receive our e-newsletter, including information on related online learning opportunities. For further information on how we process and monitor your personal data, and information about your privacy and opt-out rights, click here.