The Ether: Diversity
Casey Simple is back sharing thoughts on diversity in AI
Add bookmark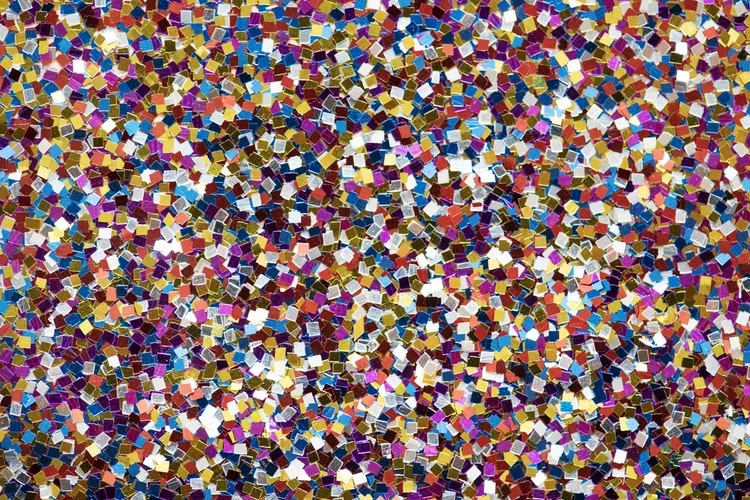
Diversity in AI
Diversity is an issue similar to ethics that we need to really be intentional about. We need to look at how we're incorporating it in our hiring of AI professionals, in our composition of AI teams, in how we're looking at the actual work that we're doing, as we're identifying data points, and as we're looking at algorithms. If we really want to infuse AI with the richness of human experience, we have to consider diversity in the teams that are doing AI.
It can be a very powerful way of learning more about the human condition and how we make decisions in what is going on in our world. But it only works if we are designing from a base of diversity. Diversity includes global point of view, culture, ethnicity, gender, educational experience, economic experience, political points of view, and more. All of those things have an impact on diversity.
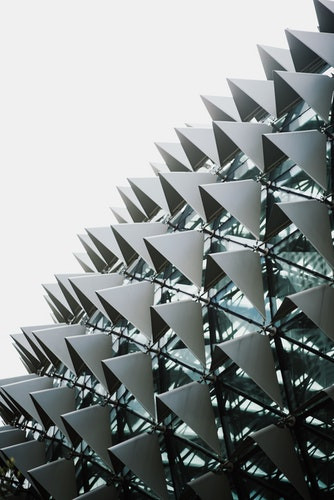
Diversity through structural change
To be intentional we have to be structural in what we're doing with our AI teams. We need to identify the fact that diversity is an important value, and therefore we need someone on our AI boards and our AI teams who is intentionally looking at diversity. It's should be a regular topic of discussion. A diversity representative should be someone who understands the implications that it has on what we're doing around AI. They could potentially be the person who's looking at data outliers. It’s an interesting possibility.
Learning from diverse data points
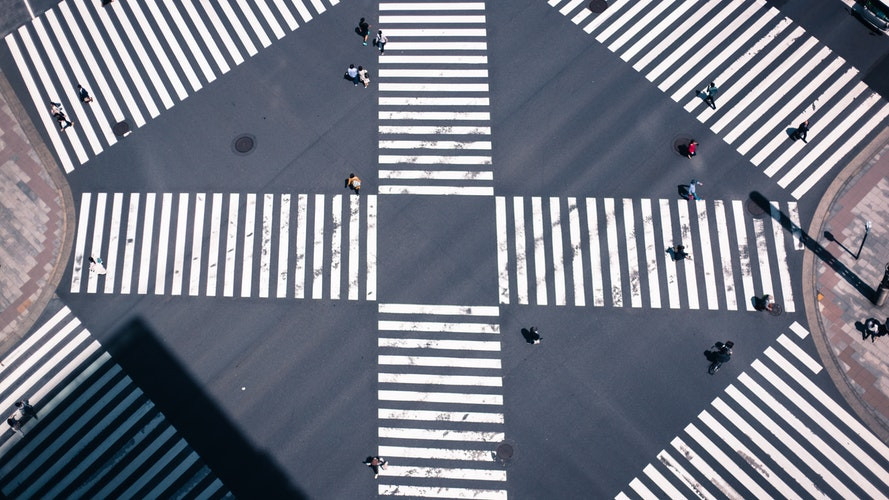
Data outliers then become important data points of learning because you have to decide: is it truly an outlier, or is it the start of a trend for a population we're not considering? The start of a trend for a set of data where we do not have diversity? Should it be inclusive as opposed to an outlier?
Those are the kinds of things that can eventually identify and potentially relieve unconscious bias in our thinking. Think of the diversity in points of view that people have around shopping, for example. Do they care more about value or total spend? Do they like something new, or do they want a repeat purchase of the same thing that they've bought in the past? Do they care about what experts think, or do they care more about what their friends and family think? Do they not care about geography, or do they care about participating in shopping locally?
And that's a simple example. Those are the kinds of things that we need to be considering when we talk about diversity for AI.
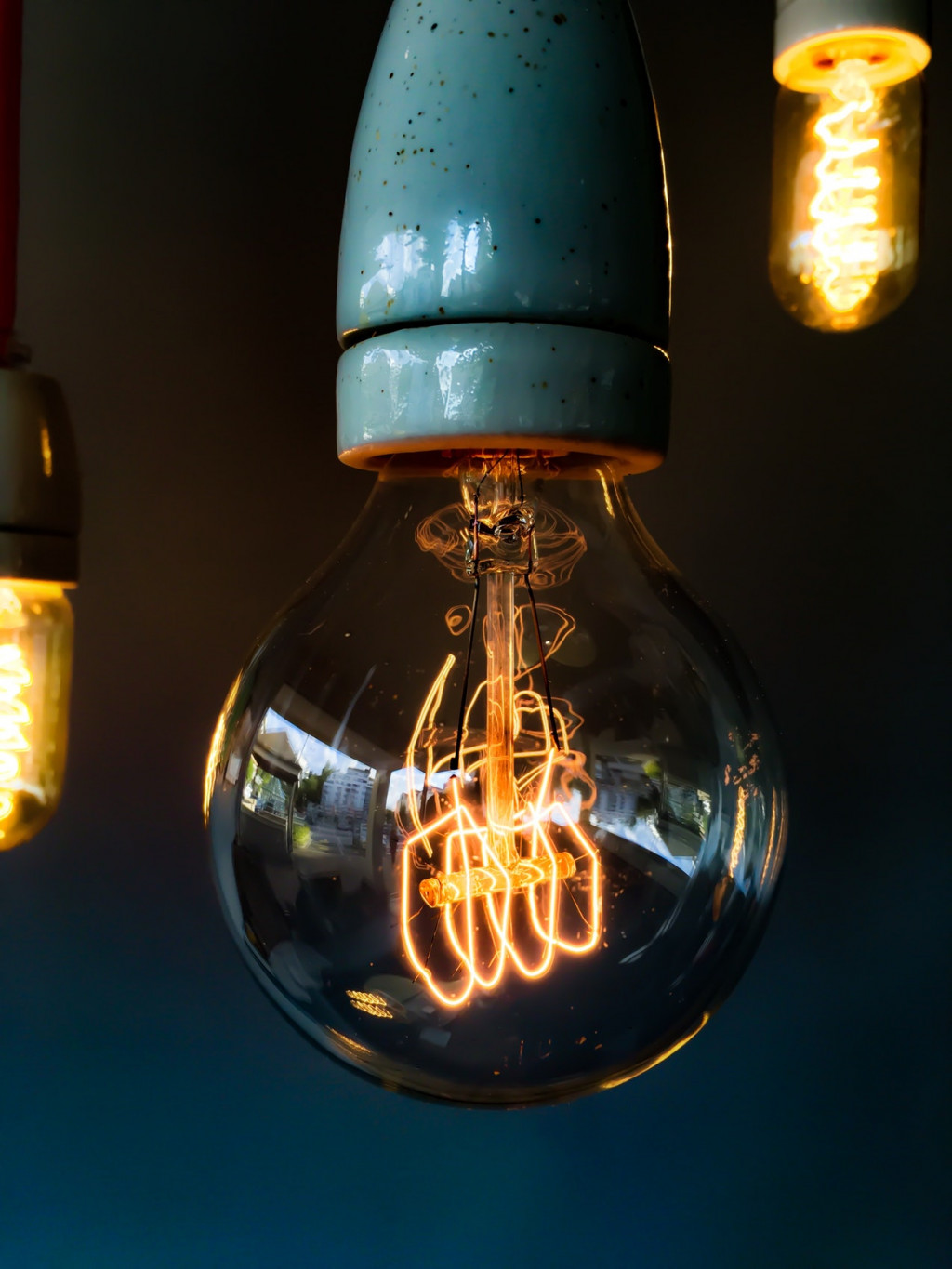
Diversity leads to innovation
The other thing we need to consider is that innovation comes from divergent thinking, and if we want true innovation, we have to have divergent thinking on our AI teams. The way you accomplish divergent thinking is to have diversity. You have to have people who think differently, who see things differently, and who have a different way of approaching things. If we really want innovation, diversity is fundamental and baseline for that.
Understanding that innovation comes from diversity also introduces the fact that the total addressable market of whatever you're offering is much larger if your data is completely inclusive, as opposed to exclusive.
Diversity in the future workforce
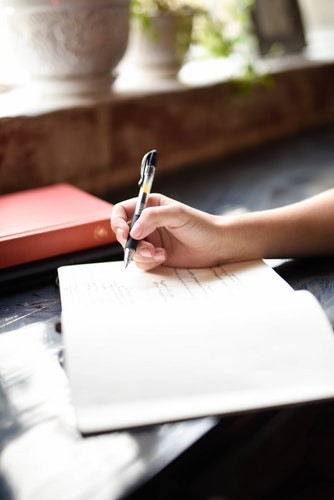
If we're really going to do this right, it has to start with education. We have to be looking at middle school and high school education, and asking: are we allowing for an interest in a diverse population in AI at a very early age? If we're not talking about it or offering it as a way of thinking and understanding, then we can't build a diverse talent pool.
It has to start early—as early as middle school, especially with regards to gender. All of the research points to this. In general, if we want to get a diverse population of students interested in educating themselves around AI, we have to start at a very early level in our educational system.