How Data Virtualization Puts Enterprise Machine Learning Programs into Production
Refining complexity in data science outputs
Add bookmark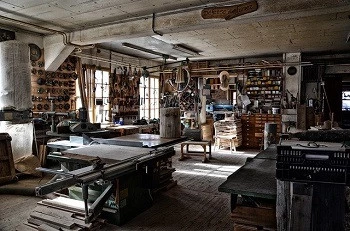
How many tools do you employ to perform data activities? The variety of data sources, the diversity in data type, the largess of data volumes and the multiple targets for analytics all result in complex solutions for data science outputs.
This session identifies Data Virtualization Benefits for AI and ML projects including:
- Reducing data exploration and analysis timeframes
- Extending and integrating capabilities to improve data scientist toolsets
- Leveraging technologies to harness large data volumes
- Expanded tools for citizen analysts
Watch the session on demand now.
Session Overview:
How Data Virtualization Puts Enterprise Machine Learning Programs into Production
Chris Day, Director, APAC Sales Engineering, Denodo
Advanced data science techniques, like machine learning, have proven an extremely useful tool to derive valuable insights from existing data. Platforms like Spark, and complex libraries for R, Python and Scala put advanced techniques at the fingertips of the data scientists. However, these data scientists spend most of their time looking for the right data and massaging it into a usable format. Data virtualization offers a new alternative to address these issues in a more efficient and agile way.
Attend this session to learn how companies can use data virtualization to:
- Create a logical architecture to make all enterprise data available for advanced analytics exercise
- Accelerate data acquisition and massaging, providing the data scientist with a powerful tool to complement their practice
- Integrate popular tools from the data science ecosystem: Spark, Python, Zeppelin, Jupyter, etc.
Watch the session on demand now.