Prediction Analysis Of Industry From Start To Future
From Start to Future Industry Analysis
Add bookmark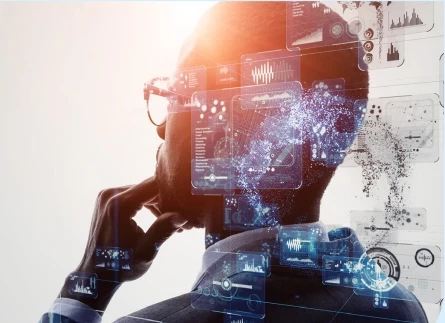
Introduction
Predictive analytics refers to using historical data, machine learning, and artificial intelligence to predict what will happen in the future. This historical data is fed into a mathematical model that considers key trends and patterns in the data. The model is then applied to current data to predict what will happen next.
The key for global corporate enterprise is to benefit from the collective intelligence presented by RPA and cognitive technologies along with human workers. Only by having technology combine with human talent can global corporate enterprise achieve scalable intelligent automation. And only with scalable intelligent automation enterprise resiliency be realized. Join that community for lessons learned at SRIA Live.Scalable RPA & Intelligent Automation Live
Using the information from predictive analytics can help companies—and business applications—suggest actions that can affect positive operational changes. Analysts can use predictive analytics to foresee if a change will help them reduce risks, improve operations, and/or increase revenue.
At its heart, predictive analytics answers the question, “What is most likely to happen based on my current data, and what can I do to change that outcome?”
As referenced in our Enterprise ML Report, machine learning has two categories:
• Classification models are used to break down large datasets into meaningful subsets. Examples are image recognition and natural language processing.
• Regression models identify trends to make predictions. Use cases are sales forecasts that take into account myriad factors from macroeconomic indicators to weather forecasts to political threats.
By reading the definition it becomes clear that ‘Predictive Analytics’ is the type of machine learning that uses regression models.
Also read: Workload Automation
Predictive analytics questions (Part I)
The answers related to predictive analytics, required by a company or enterprise executive can only be found by asking the right questions. The following are some of the crucial questions that need to be asked and analysed by any executive who is trying to understand the prospects of predictive analytics for her enterprise.
Big questions
- Is Predictive Analytics even the right way to go?
It is important to understand that ‘Predictive Analytics’ is a form of Machine Learning. Hence if you are simply trying to implement the power of ML for your company in hopes of increasing efficiency or reducing costs, then the first thing that needs to be identified is – the type of ML that’s required for the betterment of the enterprise. As mentioned in the previous section, the type of ML that uses regression models are the ones that are capable of harnessing predictive capabilities. If your enterprise generates a lot of data, and if you’re looking to find a way of using all that data to find trends or patterns that would give useful insights and predictions about your products, services or clients, then yes, ‘Predictive Analytics’ is the way to go. Furthermore, if you are - trying to attain a set of technologies that support decision-making within the enterprise focusing primarily on executives, middle management, and the analysts who support them – then it’s ‘Business Intelligence’ that you seek and not ‘Predictive Analytics’. The mandatory criteria for a technology to be termed as ‘predictive analytics’ is – future projections. If you seek technology that is capable of making models projecting ‘future’ sales and consumer product adoption, then ‘predictive analytics’ is the way to go.
- What is it that you’re looking for from your past data?
This is an important step as it sets the tone for the beginning of any project involving ML. As mentioned earlier, ML should not be treated as a ‘magical’ solution that can solve any problem. Before implementing predictive analytics through ML and investing money in it, you first have to identify exactly what aspect of your business or key performance indicator that you’re trying to predict.
- Do you have the data to answer those questions?
Is your operational system capturing the needed data? How clean is it? How far in the past do you have this data, and is that enough to learn any predictive patterns?
Predictive analytics questions (Part II)
Data & cost questions
The next set of questions that need to be asked are a general requirement for any type of ML technology and as predictive analytics is a subset of ML, these questions become quite necessary to be analysed by any executive.
• Do I have any data? Do I have enough data?
Yes, this sounds like an obvious question, but machine learning works best when you have significant amounts of data, with no signs of slowing down its accumulation. Deploying ML against a relatively modest set of data is almost like trying to start a charcoal fire with a blowtorch: Yes, you can do it, but why would you? There may be more cost-effective ways of analysing your data. (Defining a “relatively modest set” is a matter of perspective: Several years ago, “relatively modest” meant several hundred gigabytes. Today, it may mean dozens of terabytes or even several petabytes.)
• Have I thought about the total costs involved?
Also read: Enterprise Data & Business Analytics
Copious amounts of data mean outsized storage costs. While the per-gigabyte cost of storage is sharply lower than it used to be- you can still run up a sizable tab. That’s before you’ve even considered computational costs: How much data do you analyse? How often? Who does it? In many cases, a data scientist may be needed to coordinate efforts. A cost analysis of the options is required to help make an informed decision.
Three years running:
AIIA Members expect Predicative Analytics to impact their enterprise more than any other technology.
Predictive analytics questions(Part III)
Talent questions
• What are some of the options available to hire the right talent?
- Build
There are a few options available when it comes to hiring the right talent and like any other recruiting episode, it boils down to fulfilling the requirements and budget constraints of the company. If the budget permits, then the company can choose to hire Data Scientists and Machine Learning Engineers and set up its own AI department. But as mentioned in our ML Report, it’s important to keep in mind that ML engineers on average require double the salary of regular software engineers.
- Buy
The next option is to find a vendor team that specializes in providing machine learning solutions. Exploring machine learning with cloud engines is perhaps the easiest way to gain access to machine learning technology. The biggest challenge of outsourcing machine learning tasks is to align corporate limitations of sharing data with external expert assistance. Depending on the type of data you have, you may need to anonymize it in a way that it doesn’t reveal sensitive details, like customer contacts, their location, etc. You should also keep in mind that an anonymized data set doesn’t allow an analyst to enrich it by using external sources or applying his/her own understanding of a problem to build a more efficient model.
- Grow
One other option that has the potential to save a lot of money is to partner with universities. In the US, there are about a dozen Ph.D. data science programs available at universities and nearly the same number of computer science programs that are actively emphasizing data science. Another popular way to fill the skills gap is boot camps where attendees take 12-month or so courses. This option seems very promising for companies that aren’t ready to invest into hiring experienced experts, though you should always consider additional internal training to accumulate essential domain expertise.
Predicitive analytics challenges (PART I)
Implemetation challenges
Predictive analytics is a complex capability, and therefore implementing it is also complicated and comes with challenges. When companies take a traditional approach to predictive analytics (meaning they treat it like any other type of analytics), they often hit roadblocks. Predictive analytics faces numerous implementation challenges.
These challenges fall in 2 categories of technical and organizational challenges, and they are the following:
1. Technical
• Volume of required data
• Difficulty of accessing source data
• Difficulty of using the results
• Focusing on past pattern
• Difficulty of integrating predictive analytics into enterprise’s IT systems
• The data is too expensive to measure
• Low accuracy of results
2. Organisational
• Lack of recourses including budget and skills
• Lack of awareness – an understanding of how to apply predictive analytics to business problems
• Lack of in-house experts to implement the results
Predictive analytics challenges (Part II)
Turning insights onto questions
Expertise is a challenge because predictive analytics solutions are typically designed for data scientists who have deep understanding of statistical modelling, R, and Python. This is inherently limiting. In fact, most application teams can’t even begin to approach predictive analytics without first hiring a dedicated data scientist (or two or three!).
It’s not a secret that the more difficult a new technology is to use; the less likely end users are to adopt it—and predictive analytics solutions are notoriously difficult in meeting this challenge. This is because they typically live as standalone tools, which means users have to switch from their primary business application over to the predictive analytics solution in order to use it. What’s more, traditional predictive tools are hard to scale and deploy, which makes updating them a painful process.
No information is valuable in a vacuum. And that’s one of the reasons predictive analytics has fallen short in empowering end users. Often, predictive tools deliver information and insights, but they fail to let users take action. As we discussed above, if users want to act on the data, they have to jump to yet another application — ultimately wasting time and interrupting their workflow.
Predictive analytics use cases
With the understanding that Predictive Analytics is the type of machine learning that uses regression models, here are a few use cases to consider:
• Predictive Marketing recommends products and services to users based on their past behaviour.
• Targeting of website users by combining browsing behaviour within product categories and sending targeted emails for each customer segment.
• Targeting potential customers, generate leads and close sales. Identify potential high-value customers ready to make a purchase. A sales representative then contacts the customer directly and walks them through the sales process to find the perfect motorcycle.
• Matching customers to product: StitchFix is a retailer that has a unique sales model that asks users to take a style survey then uses predictive analytics to match customers with the clothes they might like. If the customer does not like the clothes they receive, they can return them for free return shipping.
• Engaging in churn prediction: Predicts what customers want and provide them the offer when they are most at risk of leaving the company.
• Identify fraudulent activities by pin pointing unusual data points: Have the model delivers a list of prospective customers at highest risk, which allows the organization to communicate with methods such as direct marketing and follow-up calls.
Also read: What is Process Mining?
Conclusion
Asking the right questions will get you the right answers for your enterprise through predictive analytics. But that’s only if you’ve focused the organization in the right direction. And that’s only if you’ve got valuable historical data and/or a comprehensive data strategy moving forward which takes into account the myriad data sets you’ll need and the associated costs of gaining and storing that data.
Even if you ask the right questions- if your talent isn’t up to the task, you will not be able to be in a position to procure the right answers. Whether it’s built, buy or grow- you must acquire the unique talent needed to get the most out of predictive analytics.
Even if you have the right talent- the enterprise must be structured to successfully implement and then gain from the insights from the action employed. Only when each of these factors is achieved can your enterprise gain value from predictive analytics.