Building Your Team To Tackle Your Unstructured Data Now
Tackle dark data by getting the right people in the right place
Add bookmark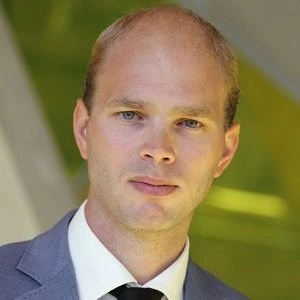
We respect your privacy, by clicking "Download Your Copy" you agree to having your details passed onto the sponsor who may promote similar products and services related to your area of interest subject to their privacy policy. You have the right to object. In addition, you will receive our e-newsletter, including information on related online learning opportunities. For further information on how we process and monitor your personal data, and information about your privacy and opt-out rights, click here.
Bob Kurpershoek
Director Innovations and Strategic Initiatives
NBCUniversal Media, LLC
Realizing the actual dark/unstructured data itself
Most companies are considering or have embarked on RPA, where best processes to robotize — with highest ROI are — high volume, standardized processes, reparative in nature and all have structured data. However the majority of your data is unstructured. It’s that unstructured data which will lead to exponential growth.
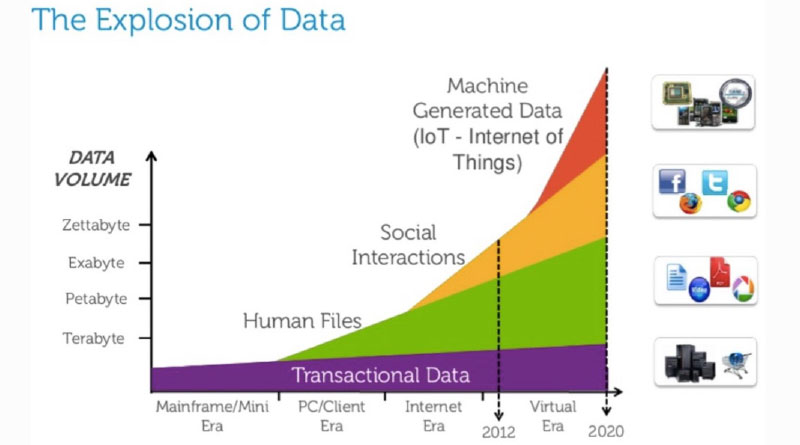
Again - most companies are making business decisions based on structured data, which is a fraction of the total amount of available data. Your unstructured data refers to information that is not organized in a pre-defined manner.
The ability to access and make sense of your unstructured data will provide remarkably valuable information, which will propel the next level of automation. If companies and/or teams can do this well, they will attain competitive advantage.
So you have a data strategy?
It’s important to look at what these types of data essentially mean, where they come from, how they are used and, most of all, how they can generate value. Currently companies are mainly looking at structured internal data sources and have not started analyzing external data sources. External data sources are unstructured. Businesses need to know how to gather this information together and build from the knowledge contained within it. Even unstructured data has to be properly organized. There is a great deal of other important information hidden in unstructured data. This is where Artificial intelligence actually comes into play — as it can deal with all of this as unstructured data, which simply cannot be dealt with by a rule basis approach.
Actually finding AI talent (which is difficult)
Organizations looking to attract AI talent are finding expertise scarce and costly. Most of the current talent in the workforce is at the big tech companies. The reality is that many companies — especially those outside tech industries — are still at the beginning of their journey.
And so, to simply remain ‘current’ we must be preparing for the future and determining our HR strategy if we want to build in-house or collaborate with external providers. In my company we value the AI intellectual capital but also want to understand the benefits. The big tech companies like Google, Amazon, and Microsoft have developed enterprise AI solutions — this makes the development of your own AI obsolete.
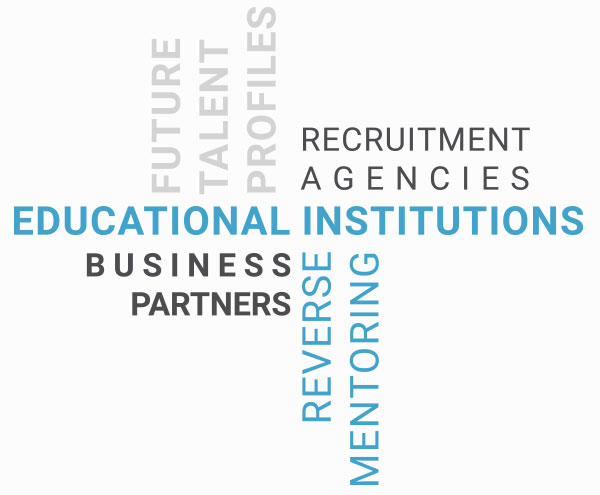
‘Big tech’ offers AI-as-a-Service such as MS Cognitive Services. If you accept their SaaS services, it may mean you do not require a heavy AI developer but rather a quality IT person/developer that can apply these solutions. But even these people are in short supply.
We have educated our HR department and business partners to define future talent profiles. Together with HR we have created a network of recruitment agencies and educational institutions. We have created reverse mentoring to ensure we find internal bottom up talent.
How familiar is the organization and how ready is the staff for this AI technology? We all require change management capabilities throughout the enterprise. Each organization must have someone who evaluates the organization’s AI maturity while simultaneously understanding the change culture. We need talent who can explain — in simple terms — what AI can do, and not do - and what that means for the current staff. This talent needs vision, network, communications skills, bridge tech and business while retaining a competitive overview.
Showing the art of the possible or demonstrating value to the enterprise:
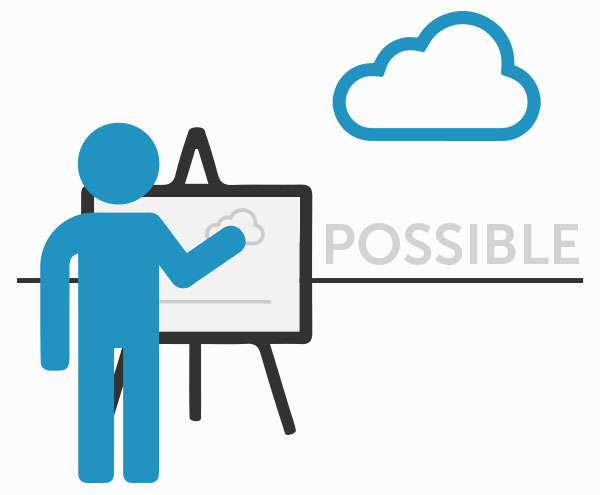
There are still very few practical AI examples. Those out there come at a cost, which makes your ROI still quite difficult in a small scale project. Similar to RPA the use of pilot programs is recommended. The same principles of RPA apply to AI - go simple, fast, low cost and with a narrow scope. Be able to show the art of the possible via a demo. Using existing AI software can be effective, as it helps your enterprise build skills and learn as it goes. The AI services of Google, Amazon and Microsoft enable small-time developers in AI, without the upfront costs or hassle-which make them a safe bet. Another way to go about this is to ask yourself, ‘can you collaborate with business development, R&D, etc. departments?’ They traditionally will focus more on customer facing parts of the business and have more advanced solutions than backend parts of the business. And if you can’t collaborate, steal with pride.
While AI is your toolkit for unstructured data, AI is defined broadly and can be deployed in many different ways. Before you do anything in this area ensure the following:
- Clearly defining how AI can solve both immediate and future problems is an imperative.
- Having a clear value proposition/business case is vital to any data-driven initiative, especially one involving the collection and management of unstructured data sets
- Marketing, customer satisfaction, healthcare and supply chain all apply
- For finance there are many opportunities:
- Advanced analytics to use unstructured data will provide more attributes to enhance knowledge based processes and sense making: credit decisions, risk analysis, budget process, better forecasting and assist with judgment calls.
- There is a real opportunity to become more forward looking — in conjunction with RPA it will mean next level of automation.
- Eventually finance will also use Conversational Interfaces, Microsoft Cognitive Services provides Language Understanding (LUIS) there could be a PoC there.
- Last but not least, try to use as much AI assistants AI in your own devices (e.g. Siri, Alexa) to trigger ideas of the art of the possible…
- You might find that AI can be used to enlighten customers and business leaders
Proving an environment that is adaptable to change:
'A digital strategy is often characterized by the application of new technologies to existing business activity and/or a focus on the enablement of new digital capabilities to their business…' (Wikipedia).
The same is applicable to AI and similar to RPA. Larger corporations of course are having the discussion of whether it should develop from IT or from business function, where it directly supports the processes. A CoE (IT or Business) is a good place to start because often they have an agile, nimble development framework and experience with PoCs.
Take the incremental approach with AI as it will be a safe environment to experiment. The expectation is also that RPA vendors will broaden their scope to AI so this will help a more easy transition. This will lead to more rapid development and higher AI tech adoption. CoEs are a good place for ideation. Again the right place to start is not in technology, but in the business cases that you want to test.