Establishing a Vision for Intelligent Automation Success: 6 Key Considerations
Intelligent Automation (IA) Roadmap Quick Guide
Add bookmark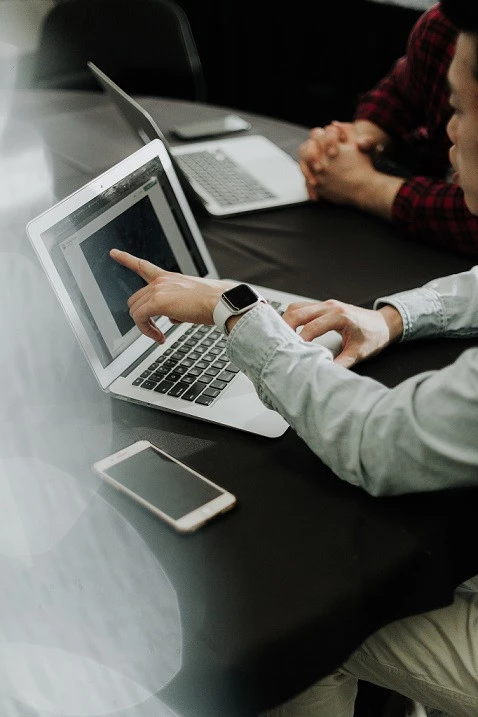
By combining Robotic Process Automation (RPA) and artificial intelligence (AI), intelligent automation (IA) systems rapidly collect, analyze, and make decisions about textual information. This enables IA to not only automate complex processes or workflows from end-to-end, but also learn, adapt and continuously improve as it goes.
However, in the world of RPA, IA and artificial intelligence, failure is common. In fact, according to a recent report by Bain & Company, almost half of automation projects fail to deliver the expected savings according. When AI is added to the equation, the stats are even worse as only 12% of AI initiatives even make it into production.
In addition, many early adopters of intelligent automation (IA) have found themselves in a bit of predicament. Though they may have enjoyed some initial success applying these tools to individual processes, in their well-intentioned but perhaps rushed attempts to implement IA first, a number of organizations have found themselves juggling disparate, poorly cobbled together automated systems. While these teams may devote massive resources to managing these complex and costly systems, real, tangible ROI likely remains elusive.
What separates the IA success stories from disaster epics is clear: organizations that cultivate a unambiguous, enterprise-wide vision and roadmap for what exactly they want to achieve strategically with IA are way more likely to reap the benefits of such initiatives than those who do not. By establishing a big-picture strategy for deployment, these organizations are better equipped to ensure all of the different components of IA -process orchestration, robotic process automation (RPA) and artificial intelligence (AI) - work together in harmony. Intelligent Automation only succeeds with an IA Vision.
The following explores the 6 key pillars for establishing a holistic, action-plan for intelligent automation (IA) implementation and maximizing the long-term value of such initiatives.
Define your business outcomes first
It sounds a bit obvious but establishing what the big-picture strategic objectives you want to achieve with IA before you begin is critical to success. The goal here is to ensure that automation strategy is completely aligned with business strategy.
To do this, Deloitte recommends you consider the following 5 things:
- Why does automation support your business needs?
- What are the benefits?
- What are the pain points being alleviated?
- What are the metrics to determine whether automation is valuable?
- What is the strategy for re-deploying existing resources after automation?
Automation CoE
A Center of Excellence (CoE) is an in-house entity that provides leadership, expertise, and best practices for a specific area of focus. In the case of Automation CoEs, as the name suggest, their key area of focus is automation.
Building an automation CoE helps reduce the risk of automation in a number of ways:
- Silos are the enemy of IA. Automation CoEs helps break them down through the creation of one, centralize and cross-functional automation team
- It enables the development of one shared vision or approach to automation implementation thus the creation of standardized automation policies and procedures
- It helps avoid redundant or duplicate deployments
- Because CoEs are often able to deploy automation more efficiently, it reduces time to market
- Increases business agility as automation efforts are more easily scaled up or down
- It helps leverage economies of scale
- As one central team oversees executions, it helps ensure data streams and systems are fully integrated
According to automation experts Workfusion, automation CoEs typically fall into one of 4 categories:
- Automation Center of Expertise. This COE model focuses on the training and enablement of automation development and implementation teams. Other goals include the development and advancement of RPA and IA best practices and building automation skills across teams.
- Automation Center of Excellence. This COE model focuses on methodology, best practices, and integration with re-engineering projects.
- Automation Factory. An Automation Factory delivers quick and efficient automation results and usually deals with process-level automation.
- Automation Community. Unlike the other center of excellence models, this model is organized as a community sharing information, expertise, and best practices across lines of business. The focus of this model is on tactical and departmental projects.
In some organizations, the automation CoE is responsible for managing the entire automation lifecycle from vision to operationalization. However, especially in larger organizations, the centralized (or enterprise-wide) automation CoE is only responsible for creating the overarching automation framework or strategy. Individual CoEs within business units then use this framework to deploy their own automation frameworks. This is known as federated deployment.
For example, AbbVie's Robotics Process Automation CoE Lead, Ana Rudman recently explained in a recent interview that, because Abbvie is a large, global company, they chose to go with a centralized model with federated deployment. “This means we have one common enterprise RPA platform, a centralized demand intake FIT assessment process, development standards best practices, and most importantly, governance around reusable assets that enable continued automation agility.”
Process Analysis, Prioritization & Excellence
Organizations are more than just a hodgepodge of disconnected systems and services, they’re complex ecosystems of interconnected processes, value chains, capabilities, resources and much more. A delay or inefficiency in one area can affect all others. For example, process challenges in finance & accounting can impact customer experience if returns are delayed or employee experience if paychecks go out late.
In order for solutions as costly and labor intensive as IA to really achieve any meaningful ROI, it must be scalable across the entire enterprise. The only way to ensure this is by integrating people, processes and technology.
Process mapping, process mining and process discovery are the three most common approaches for doing this.
- Process discovery is “a collection of tools and techniques used to define, map and analyze an organization’s existing business processes.” This approach helps companies prioritize which processes to automate first as well as identify and re-engineer broken processes so they can be potentially automated down the line.
- Process mapping is the practice of distinguishing the relationship between processes and other business artifacts (i.e. strategies, business capabilities, outcomes, etc.). Using process mapping software, process maps show a series of events that produce an end result, typically in the form of a flowchart.
- Process Mining refers to the discovery, monitoring and improving of real processes (i.e., not assumed processes) by extracting readily available knowledge from the event logs of information systems.
The goal is to build a comprehensive, 360-view that not only documents and lays out all business processes, but shows how they interconnect as well. As Everest Group explains, “A 360-degree view involves designing experiences/interfaces not only at the end-customer touchpoints, but across all the key constituents of a business ecosystem, including employees, partners, suppliers, and regulators.”
Image sourced from "Everest Group: 360-degree Enterprise Automation Playbook," https://www.uipath.com/resources/automation-analyst-reports/360-degree-enterprise-automation-playbook
Data Management & Governance
Intelligent automation is only as good as the data that powers it. As RPA bots and artificial intelligence require massive amounts of data to train on, implementing a robust data management framework and governance program is mandatory for IA success.
As one writer put it, “Starting an AI project without checking your data first is like building an F-1 race car without understanding what quality, type, specification, or sustainability of fuel you want to use.”
According to the Data Governance Institute, data governance is "a system of decision rights and accountabilities for information-related processes, executed according to agreed-upon models which describe who can take what actions with what information, and when, under what circumstances, using what methods."
Essentially a collection of standardized rules, technologies and processes that dictate how data is collected, handled and stored within an organization. It also encompasses other data management concepts such as Data Stewardship, Data Quality, and Data Security.
Most successful data governance programs aim to strike a balance between data collection practices, privacy mandates and data security. Not only does this help protect against data loss and errors, but the potential misuse of sensitive information (i.e. customer data) as well.
The DGI Data Governance Framework © The Data Governance Institute https://profisee.com/data-governance-what-why-how-who/
Don’t Forget The Human Factor
No matter the potential business value intelligent automation brings to the table, an organization’s people is still its #1 asset. With this in mind, it’s critical your IA strategy accounts for people-related considerations: closing the AI skills gap and change management.
According to Deloitte’s 2019 report, Automation with Intelligence, 14% of respondents listed “availability and capability of talent” as their top barrier to IA adoption, making it the third most popular selection. In a follow up 2020 study, 23% of advanced AI adopters said they’re experiencing “major or extreme” AI talent shortages.
The fact is many organizations simply don’t possess the skilled, qualified talent or in-house expertise necessary to make intelligent automation work. Not only that, but the extreme lack of diversity in terms of who writes AI code (mostly white, heterosexual men) has resulted in AI bias. And the problem is getting worse.
According to Gartner, “By 2022, 85% of AI projects will deliver erroneous outcomes due to bias in data, algorithms, or the teams responsible for managing them. This is not just a problem for gender inequality – it also undermines the usefulness of AI”
In order to overcome these challenges, companies need to develop long-term talent management strategies to ensure they have the right talent in place at the right time. This not only means developing an action plan for hiring more data scientists and software engineers, but also ensuring that the millions of workers displaced each year due to automation are trained to do something else.
Furthermore, having a robust change management plan in place helps ensure people not only know how to use new IA tools, but they embrace them to their full capacity as well. Considering an estimated 37% of workers are worried about losing their jobs due to automation, a sound change management plan helps organizations ease these fears and prove to employees how IA will actually enhance their work experience, not hinder it.
Monitoring IA & Looking Ahead
Last but not least, establishing a clear understanding of how to capture value both in the near and long terms. This should include both quantitative and qualitative measures such as:
- Operating cost savings - reductions in FTEs and other overhead costs
- Increased profitability - a topline boost due to things like decreased revenue leakage and customer churn
- Increased speed and efficiency - how much faster does IA complete the work compared to humans?
- Improved customer experience - faster and more streamlined service
- Improved employee experience - IA can helps increase worker productivity while also reducing administrative burden
- Advanced Data Management & Analytics - IA not only enables improved data management & security, it also generates massive amounts of high value data. How can this data be used not only to improve operational efficiency, but boost revenue?
- Accelerating Innovation - Not only does AI boost innovation by reducing administrative burden, it also helps companies deliver new, better services
It’s also important to note that ML and AI models tend to degrade over time due to a phenomenon known as “concept drift.” As a result, AI/ML models and RPA bots need to constantly be monitored for accuracy and frequently retrained. The costs and efforts associated with this should also be incorporated into your long-term strategic plans as well.
Please take 5 minutes to tell us about your digital transformation story for our 2021 Annual Industry Report:
Create your own user feedback survey
Can't view the above survey? Access it here: https://www.surveymonkey.com/r/FBS6G2B
We respect your privacy, by completing this survey and clicking "Submit" you agree to having your details passed onto the sponsor who may promote similar products and services related to your area of interest subject to their privacy policy. You have the right to object. In addition, you will receive our e-newsletter, including information on related online learning opportunities. For further information on how we process and monitor your personal data, and information about your privacy and opt-out rights, click here.